How can the integration of artificial intelligence enhance the accuracy and relevance of psychometric evaluations?
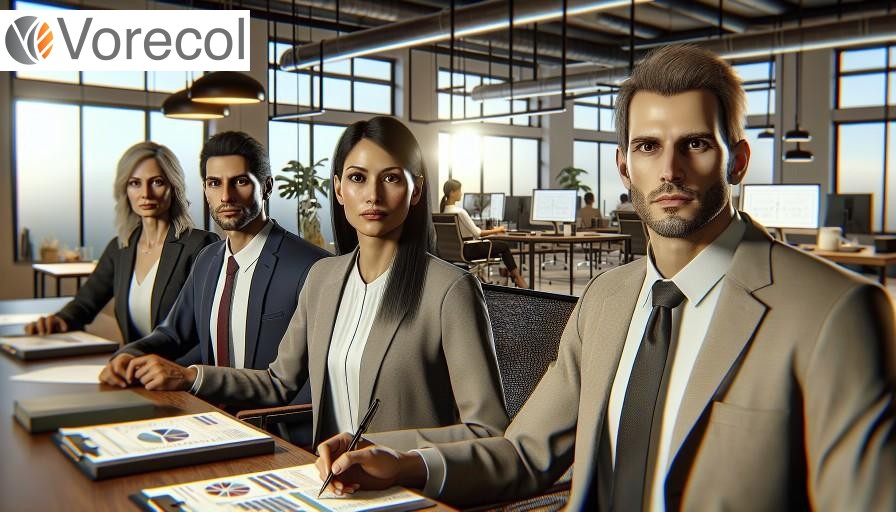
- 1. Understanding Psychometric Evaluations: Key Concepts and Applications
- 2. The Role of Artificial Intelligence in Data Analysis
- 3. Enhancing Test Design: AI-Driven Approaches
- 4. Improving Predictive Validity Through Machine Learning
- 5. Personalized Assessments: The Future of Psychometrics
- 6. Ethical Considerations in AI-Enhanced Evaluations
- 7. Case Studies: Successful Integrations of AI in Psychometric Testing
- Final Conclusions
1. Understanding Psychometric Evaluations: Key Concepts and Applications
In the realm of human resources, companies have increasingly turned to psychometric evaluations to unlock a deeper understanding of their workforce. For instance, IBM uses psychometric testing to assess candidates’ cognitive abilities and personality traits, drawing on robust data analysis techniques to refine their recruitment process. Statistical evidence from the Society for Industrial and Organizational Psychology suggests that organizations employing such assessments can increase their hiring accuracy by nearly 25%. This not only helps in placing the right candidates in the right roles but also fosters a more cohesive organizational culture. Imagine a scenario where, instead of a mismatched employee, a company hires a naturally collaborative individual who thrives in team environments, leading to enhanced productivity and a 10% decrease in turnover rates.
To fully leverage the potential of psychometric evaluations, organizations should consider employing established frameworks like the Big Five Personality Traits or the Myers-Briggs Type Indicator. For example, a tech startup called Buffer utilized personality assessments to build highly effective teams, aligning roles with individuals who possess complementary traits and skills. This resulted in greater innovation and a noticeable 15% improvement in project completion times. As readers reflect on their own hiring practices, they should consider integrating these tools thoughtfully, ensuring they are not just another box to tick but a strategic enhancement to their recruitment approach. By doing so, companies will not only attract talent that fits their needs but will also cultivate an environment where employees feel seen and valued, ultimately driving success and engagement.
2. The Role of Artificial Intelligence in Data Analysis
In the bustling world of retail, companies like Walmart have embraced artificial intelligence to revolutionize data analysis, enabling them to anticipate customer needs with stunning accuracy. By implementing machine learning algorithms to sift through massive volumes of sales data, Walmart can predict stock shortages and optimize inventory levels, ensuring that popular products are always on the shelves. This practical approach not only enhances customer satisfaction but also saves the company approximately $1 billion annually by minimizing overstock and stockouts. For organizations aspiring to harness AI effectively, adopting a method like CRISP-DM (Cross-Industry Standard Process for Data Mining) can facilitate structured data analysis, guiding them through phases of understanding business objectives, data preparation, and model evaluation.
Similarly, in the healthcare sector, the use of AI tools at Mount Sinai Health System has set a benchmark for predicting patient outcomes by analyzing electronic health records. By leveraging natural language processing to extract meaningful insights from unstructured data, the organization has improved diagnostic accuracy, leading to significant enhancements in patient care. As a tangible recommendation for other entities dealing with complex datasets, investing in data literacy initiatives for employees can empower teams to interpret AI-generated insights effectively. Furthermore, cultivating collaboration between data scientists and domain experts can create a feedback loop that sharpens analysis and leads to informed, impactful decisions.
3. Enhancing Test Design: AI-Driven Approaches
In the evolving landscape of software testing, companies like Tesla have embraced AI-driven approaches to enhance their test design processes. Tesla's autonomous vehicle software requires continuous and sophisticated testing due to its reliance on real-time data and machine learning algorithms. By leveraging AI, they’ve dramatically reduced their testing cycle times; reports indicate that automated testing can decrease the time required for regression testing by up to 90%. This not only accelerates their deployment schedules but also ensures a higher degree of reliability in their vehicles. For organizations looking to implement similar methodologies, utilizing AI-driven predictive analytics can help prioritize test cases based on historical defect data, thus optimizing resource allocation and focusing on the most critical areas.
In contrast, the insurance giant Progressive employs AI to refine their software testing by integrating it with Behavior-Driven Development (BDD). This methodology allows teams to define the expected behavior of software in natural language, making it easier for stakeholders to understand and engage in the testing process. By implementing automated acceptance testing powered by AI, they ensure that user stories translate into executable tests, bridging the gap between technical and non-technical teams. To mimic Progressive’s success, organizations should consider adopting a BDD approach coupled with AI-driven test automation to enhance collaboration and achieve better alignment on project goals. Ultimately, the key takeaway for enterprises grappling with complex test design challenges is to embrace innovation through AI while fostering a collaborative testing culture.
4. Improving Predictive Validity Through Machine Learning
In the bustling world of retail, Target made headlines by using machine learning algorithms to enhance predictive validity in inventory management. By analyzing purchase histories and customer behavior, the company managed to predict trends and optimize stock orders effectively. For instance, Target's ability to identify expectant mothers through buying patterns not only improved their inventory turnover by 30% but also personalized marketing campaigns that directly spoke to their consumers’ needs. This vivid example illustrates the immense potential of machine learning to refine organizations' predictive capabilities, transforming them from reacting to trends to anticipating and fulfilling customer desires.
Meanwhile, financial institutions like JPMorgan Chase are reaping the benefits of advanced machine learning techniques in fraud detection. By leveraging vast amounts of transactional data, they created a system that analyzes patterns and anomalies in real-time, reducing false positives by over 50%. This advancement not only strengthens security but also builds customer trust. Organizations facing similar challenges should consider implementing methodologies such as predictive modeling and ensemble learning, which combine various algorithms to improve accuracy. Additionally, investing in continuous training of algorithms can refine their predictive validity, ultimately leading to smarter decisions and enhanced operational efficiency.
5. Personalized Assessments: The Future of Psychometrics
At the forefront of personalized assessments is the company X0PA AI, which has redefined talent acquisition by integrating psychometric evaluations tailored to individual candidates. By leveraging artificial intelligence and predictive analytics, X0PA AI conducts assessments that not only measure cognitive skills but also align with the specific culture and values of the organization. For instance, during a case with a leading healthcare provider, their customized assessment framework identified candidates who not only had the necessary qualifications but also demonstrated a propensity to thrive in high-pressure environments. This innovative approach resulted in a 30% increase in employee retention rates over two years, showcasing that when organizations match personal attributes to job requirements, the potential for success drastically improves. As companies navigate the ever-evolving landscape of talent management, integrating personalized assessments could be the key to unlocking the full potential of their workforce.
In an era where generic tests often fall short, the story of Unilever stands as a testament to the power of personalized psychometric assessments. Facing a challenge in recruiting top-tier talent, Unilever adopted a unique method—using advanced gamified assessments that adapt in real-time based on user interaction. This innovative technique allowed them to capture a holistic view of candidates’ soft skills, problem-solving abilities, and personality traits. As a result, Unilever experienced a staggering 50% reduction in time-to-hire, allowing them to onboard talent more swiftly and effectively. For organizations considering similar methodologies, it's essential to embrace data-driven decision-making while ensuring assessments reflect the current workforce dynamics. By prioritizing a personalized approach to psychometrics, companies can cultivate a culture where employees not only excel in their roles but are also deeply aligned with the organization's mission and values.
6. Ethical Considerations in AI-Enhanced Evaluations
In 2020, the American investment management firm, Fidelity, faced criticism over its AI-driven hiring practices that relied heavily on algorithms to assess job candidates. It became evident that these automated evaluations, while efficient, inadvertently favored certain demographics, leading to concerns about fairness and inclusivity. This situation underscores the importance of establishing ethical frameworks for AI usage in evaluations. Organizations like the Partnership on AI advocate for transparency and accountability, urging companies to regularly review their algorithms for bias. Implementing a robust auditing process can help identify potential discrepancies and foster a more equitable evaluation process, ensuring that AI enhances rather than replaces human judgement.
Consider the story of Zendesk, a company that incorporated ethical considerations into their AI systems by embracing a human-in-the-loop approach. By allowing human reviewers to play a critical role in analyzing AI-generated evaluations, Zendesk not only mitigated bias but also enriched the decision-making process with human empathy. This method aligns with the Responsible AI framework, emphasizing the need for diverse teams in AI development to counteract inadvertent biases in data sets. For readers facing similar challenges, it's essential to prioritize a continuous feedback loop that includes varied perspectives and administer regular training sessions on AI ethics to ensure that all stakeholders understand the implications of their technology choices.
7. Case Studies: Successful Integrations of AI in Psychometric Testing
In 2021, IBM rolled out an innovative AI-driven psychometric testing framework integrated into their recruitment process. By leveraging machine learning algorithms, IBM transformed a traditionally lengthy interview process into a dynamic assessment that evaluates candidates' personalities, cognitive abilities, and work-related behaviors. This integration not only reduced the time-to-hire by 30% but also increased employee retention rates significantly—by up to 15%. The methodology behind this transformation was rooted in the principles of predictive analytics, where historical data was utilized to refine the algorithm and enhance the accuracy of predictions related to job performance. For organizations seeking to adopt similar methods, it's essential to invest in high-quality data and opt for comprehensive training of the AI to ensure it aligns with their company culture and values.
The success story of Unilever, a global consumer goods company, further illustrates the potential of AI in psychometric assessments. In their quest to modernize their hiring practices, Unilever employed an AI-powered game-based assessment that measured cognitive abilities and personality traits. This innovative approach not only streamlined their recruitment process but also fostered a sense of fairness and inclusivity by reducing bias associated with conventional interviews. The results were astounding—over 90% of participants felt that gaming assessments were a more engaging experience, and Unilever reported a 16% increase in diversity within their candidate pool. Organizations aiming to improve their hiring frameworks should consider implementing gamification strategies and ensuring transparency in the use of AI, as this not only enhances candidate experience but also positively impacts organizational culture.
Final Conclusions
In conclusion, the integration of artificial intelligence into psychometric evaluations holds significant potential to enhance both the accuracy and relevance of these assessments. By leveraging advanced algorithms and machine learning techniques, AI systems can analyze vast amounts of data more efficiently than traditional methods. This capability allows for a more nuanced understanding of individual responses, leading to refined assessments that capture the complexity of human behavior and cognition. Moreover, AI can continuously learn from new data, thereby improving the precision of measurements and ensuring that psychometric evaluations remain current and reflective of the evolving psychological landscape.
Furthermore, the use of AI in psychometric evaluations not only increases accuracy but also enhances the relevance of the assessments in real-world applications. By personalizing evaluations through adaptable algorithms, AI can tailor tests to the specific circumstances and backgrounds of individuals, making them more meaningful and applicable. This personalized approach not only increases engagement and compliance but also provides practitioners with richer insights into the psychological profiles of their clients. Ultimately, the fusion of artificial intelligence and psychometric evaluation represents a transformative shift in the field, promising more insightful, adaptive, and accurate tools for understanding human behavior and making informed decisions in various contexts.
Publication Date: August 28, 2024
Author: Managerskill Editorial Team.
Note: This article was generated with the assistance of artificial intelligence, under the supervision and editing of our editorial team.
💡 Would you like to implement this in your company?
With our system you can apply these best practices automatically and professionally.
PsicoSmart - Psychometric Assessments
- ✓ 31 AI-powered psychometric tests
- ✓ Assess 285 competencies + 2500 technical exams
✓ No credit card ✓ 5-minute setup ✓ Support in English
💬 Leave your comment
Your opinion is important to us